LLM in Medical Devices, everything you need to know.
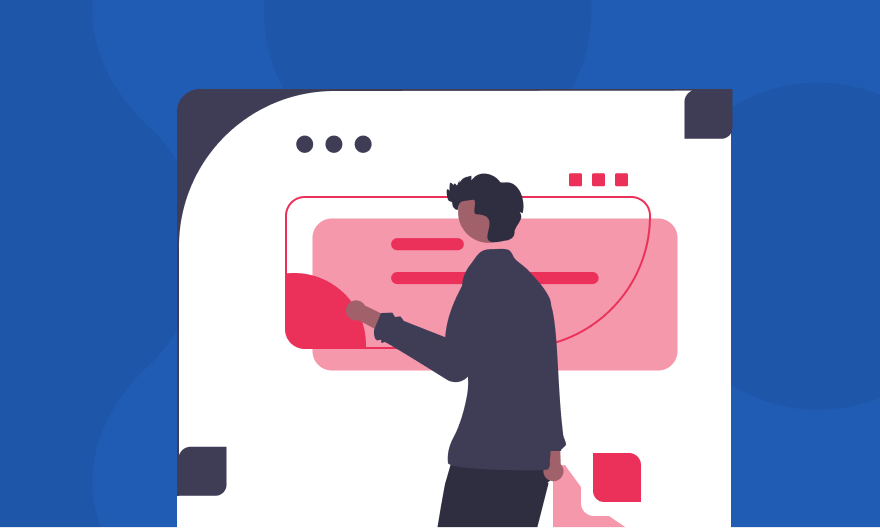
Adoption of technology is progressing at an unprecedented fast pace. It may not seem that way when living our everyday lives, in practice the speed is intimidating to say the least. When mobile phones were first brought onto the market it took around 16 years to obtain 100 million users. In 2004 when Facebook had launched it took 4,5 years to reach the same number, and WhatsApp 3,5 years after its launch in 2009. Then, a staggering 2 months for ChatGPT after its launch in 2022 (and only 5 days for Threads after its launch in 2023). It is not complex to understand that these technologies have the potential to disrupt societies and industries in a very short time.
Large Language Models (LLM)s, such as the one used in ChatGPT, use Artificial Intelligence (AI), and are trained on billions of words derived from books, articles, and other internet-based contents. LLMs utilize neural network architectures which leverage deep-learning to represent the complicated associative relationships between words as they are used in the training dataset of text-based content. In 2023, an update to ChatGPT allowed it to attain passing level performance in the United States Medical Licensing Examinations, and there have been suggestions that AI applications may be ready for use in clinical, educational, or research settings.
It is therefore no surprise that diverse applications of LLMs have appeared for general use and within the healthcare domain. Within healthcare this includes LLMs which can facilitate operational tasks without a direct medical purpose, such as summarizing clinical documentation; creating discharge summaries; generating clinic, operation, and procedure notes; obtaining insurance pre-authorization and those summarizing research papers. LLMs can further assist physicians tasks which are directly associated with medical intended purpose in, for example, diagnosing conditions based on medical records, images, laboratory results, and suggest treatment options or plans.
In this blogpost we aim to outline how LLMs and other Generative AI Systems have the potential to aid the healthcare industry, how LLMs and the like walk a thin line between different legislative frameworks, and where potential gaps in those frameworks may exist.
Large Language Models explained
Before diving into the domain of Large Language Models, it is useful to place them into a larger context. The field of Artificial Intelligence has been slowly evolving since 1967, and has seen an enormous increase in development speed recently. The first developments of neural networks already date back to the late 1950’s and one of the first successful learning AI systems was published in 1967 (i.e. the development of a nearest neighbor algorithm). Since then various advances have been made, such as the publication of a convolutional neural network architecture and backpropagation in 1980 and the development of commercially affordable GPU’s (Graphic Processing Units) in the early 2000’s which greatly increased computational power. The availability of the GPU’s quickly increased the use of machine learning algorithms and consequently deep-learning algorithms. Development was slow due to deep-learning algorithms requiring sometimes as much as even 300,000 fold increase in the amount of computational power, hence their need for affordable GPU’s to become available.
Within the overview below an overview of Artificial Intelligence techniques is presented as available today and where they fit in within the field of AI.
When ChatGPT was launched in 2022, it again introduced significant advances in the field of Artificial Intelligence. LLM are a form of General Purpose AI systems, which are based on the same principles as deep-learning. deep-learning makes use of ‘neural networks’ which include a number of small mathematical functions, also referred to as neurons. Each neuron is capable of calculating outputs based on inputs. Neurons are interconnected, and the strength of connections are determined based on numerical weights. The larger the number of neurons, the bigger the complexity of the system, where large language models typically include millions of neurons with many hundreds of billions of connections between them, and each connection having its own weight.
LLMs utilize a unique neural network architecture called the ‘transformer’. This architecture is optimized for processing and generating sequential data, such as text. Unlike traditional neural networks, transformers use a self-attention mechanism that allows them to focus on different parts of the input sequence, enabling a more nuanced understanding of context. This attention mechanism strengthens the connections between relevant parts of the data, facilitating better comprehension and generation of language. Modern LLMs can have hundreds of billions of parameters, with their weights requiring substantial storage capacity, often measured in hundreds of gigabytes.
Each weight and each of the neurons represent a mathematical formula that is calculated for each word (or, in some cases, a part of a word) that is provided to the model for its input, and for each word (or part of a word) that it generates as its output. Small words or parts of words are referred to as tokens. Large Language Models break down prompts, i.e. the user generated search term or request to the LLM, into these tokens. On average, a token is ⅘ of a word. Based on the input tokens, the LLM generates a response that sounds right based on the immense volume of text that it consumed during its training. Importantly, it is not looking up anything about the query. It does not have any memory wherein it can search for the words within the prompt. Instead, it newly generates each token of output text after which it performs the computation again, generating a token that has the highest probability of sounding right.
Concerns with LLM
The technology is promising, and the text generated by these models are not necessarily intended to provide factually accurate results, instead these models and their strengths are to generate text which reads like human-written text and that sounds right. Such text will often also be right, but not always. The technology, as is similarly true for other ‘learning’ AI technology, is subject to issues such as bias, privacy and security concerns. And at the same time is subject to generating incorrect information (referred to as ‘hallucinations’ or ‘fabrications’), where hallucinations can refer to information which is contextually implausible, inconsistent with the real world, and unfaithful to the input. Other risks include the loss of information when text is processed by LLMs, e.g. when using an LLM to summarize or convert information. More challenges exist with the use of LLMs, of which a useful and extensive overview is provided here.
LLM in healthcare
Since the publication of ChatGPT in 2022, an enormous increase in scientific papers around the use of large language models in healthcare can be observed. A quick search on Pubmed results in a single paper being published in 2022 up to 80 in 2023 and a total of 124 in the first 6 months of 2024. Hence, it cannot be surprising that there are potential use cases for LLM within healthcare.
Accurate interpretation of spoken language is one of the most critical factors influencing success of communication. Within healthcare written text is used for a lot of communication between medical professionals about patients. A lack of clarity in patient reports has been reported to correlate with inferior quality of patient care and inefficient communication between healthcare providers results in a substantial economic burden for clinical institutions and healthcare systems. LLMs can play an important role in improving communication and lowering the burden on the healthcare system. In the Netherlands one of the largest teaching hospitals (the Amsterdam UMC) has recently engaged with two other hospitals in the use of ChatGPT to answer questions posed by patients in a collaboration with Epic. In the trial, physicians receive an automatically generated response they can return to a patient. In the response, the physician needs to review and as needed alter the text before submitting the information back to a patient. The program seems to receive positive feedback and is suggested to reduce administrative burden.
The opportunities of LLMs are, as similarly demonstrated for deep-learning systems over the recent years, very broad. In a recent article published by Meskó and Topol, the authors present (in Figure 1) an in-depth assessment of potential use cases of LLM in the healthcare environment.
Figure 1. Overview of potential medical use-cases for LLM’s
Whilst highlighting the opportunities with LLMs, the authors also call for regulating these systems, to ensure that there are regulatory guardrails in place to bring such systems onto the market in a safe manner, and suggest such regulations should also address non-text generative systems based on sound and video interactions.
Regulating LLMs and Generative AI
On the 2nd of August, the AI Act will, after much anticipation, enter into force. It aims to protect fundamental rights, democracy, the rule of law and environmental sustainability from high-risk AI. The AI Act (2024/1689) is based on the same New Legislative Framework (NLF) principles as the Medical Device Regulation (2017/745) and the In-Vitro Diagnostics regulation (2017/746). It is intended to fill the gap for:
AI systems that currently are not in the (product) regulated space (e.g. educational tools), where the AI Act will be the parent product legislation; and
AI systems that are already regulated under NLF, however where that legislation does not address AI specific concerns and where the AI Act is supplemental legislation to be applied in addition to the parent product legislation (e.g. the MDR or IVDR).
In other words, if a LLM is embedded in a medical device, it is primarily regulated through the MDR and or IVDR, additional requirements set out in the AI Act would apply. If the LLM is not embedded in a medical device (or another product legislation), the AI Act would apply as a stand-alone legislative framework.
LLM’s in the context of MDR / IVDR
Although for those working in the medical device industry this is common knowledge, the first assessment to determine whether a product which incorporates a LLM is a medical device, is to assess whether it fits within the definition of a medical device. As for any product, the central question to be answered is:
“Does the product fulfill a medical intended purpose?’
To answer this question, the manufacturer should assess per Article 2 of the Medical Device Regulation (2017/745), whether the device performs an action which could be interpreted as an action to e.g. diagnosis, prevention, monitoring, of disease etc or provides information by means of in-vitro examination of specimens. If the answer is yes, then the product should primarily be regulated as a medical device, unless the software performs an action or set of actions that are no more than storage, archival, communication, simple search, lossless compression (as explained in MDCG 2019-11), in which case the MDR or IVDR do not apply.
This step is crucial in the assessment of LLM’s and in the further regulation of the LLM under the AI Act since there is an interplay between the MDR and the AI Act, where most medical devices that use AI are automatically bumped into the High-Risk category in the AI Act.
As a practical example, an LLM intended to summarize notes made by a physician as part of a patient anamnesis, where no conclusions are drawn by the LLM that may be interpreted as (an aid in) diagnosis, prevention, monitoring of disease etc, the LLM consequently does not qualify as a Medical Device. Even though such an LLM is capable of adversely affecting a patient's health. It is for good reason that Epic in their trial product decided to ensure the doctor always reviews and confirms the response to the patient before sending it back. For example, missing information or fabricated misinformation can directly cause harm to a patient. Consider the LLM noting down an incorrect dose of medication to be provided in a response to the patient. Providers of such systems should further be wary of the risk of automation bias (‘the AI seems to do a good job in responding to patients, I’ll just hit ‘respond to patient’ without reading the response in detail’). Over reliance on LLM’s may on a longer term further lead to healthcare provider’s skills diminishing over time, where in critical areas they gain fewer expertise due to reduced practice.
If the LLM in the product does qualify as a medical device, e.g. it performs an action that contributes to the diagnosis, prevention, monitoring of disease etc, then it will most likely be considered a Class IIa medical device (or higher) in line with Rule 11 of Annex VIII, or a high risk under the IVDR (class B, C or D). Such devices require conformity assessment through a Notified Body, and consequently per Article 6(1) of the AI Act will be considered High-Risk AI if the AI System is either a safety component of a medical device, or the AI system is considered to be the medical device itself (e.g. it directly contributes to meeting the device’s intended purpose, further guidance is still pending with the European Commission). This means demonstrating compliance with the requirements set out in the MDR or IVDR and the AI Act Chapter III.
LLM as healthcare software
If the LLM does not perform an action as defined in Article 2 (1) of the MDR, again, contributing to diagnosis, prevention, monitoring or disease etc, then it is consequently not considered a ‘Medical Device’, and MDR and or IVDR do not apply. Consequently, the provider of the AI System should consider whether the AI Act applies.
The manufacturer should assess whether the AI System may be:
Prohibited AI, per Chapter II and Article 5
High-Risk AI, per Chapter III, Article 6
General Purpose AI, per Chapter V
And if none of the above applies, whether transparency requirements per Article 50 apply. For the purpose of this blogpost, we won’t discuss prohibited AI practices.
LLM as High-Risk AI
High-Risk AI, per Article 6 shall be considered High-Risk if they are covered by the legislation under Annex I and require under that legislation to undergo conformity assessment (e.g. medical Devices), OR when they are covered under Annex III.
The list provided under Annex III is extensive, however, let’s call out some examples that may apply to healthcare:
Biometrics - Where AI systems are intended to recognise emotions (1(c))
Critical Infrastructure - Where AI systems are intended to be used as safety components in critical infrastructure (2)
Essential public health services - AI evaluating and classifying the dispatch of emergency services or emergency healthcare patient triage (5(d))
Based on the above, we can conclude that basic LLM to summarize or convert healthcare data, falls mostly outside of the scope of the list of Annex III above. Consequently, such AI does not seem to be considered High-Risk AI.
General Purpose AI
To better understand General Purpose AI (GPAI) and GPAI Systems, the definitions are explained below.
‘general-purpose AI model’ means an AI model, including where such an AI model is trained with a large amount of data using self-supervision at scale, that displays significant generality and is capable of competently performing a wide range of distinct tasks regardless of the way the model is placed on the market and that can be integrated into a variety of downstream systems or applications, except AI models that are used for research, development or prototyping activities before they are placed on the market;
Most LLM will be based on a GPAI model which is made publicly available, e.g. the open-source models. These systems can generate text to any data incorporated into the training data of these models. The requirements applicable to providers of GPAI models include the set up of technical documentation, and making the documentation available to parties implementing the GPAI models into their AI Systems (GPAI Systems), unless when being distributed as an open-source GPAI model. Additional requirements apply for GPAI models which introduce systemic risk.
GPAI models may be integrated into downstream systems, where the GPAI becomes a GPAI System:
‘general-purpose AI system’ means an AI system which is based on a general-purpose AI model and which has the capability to serve a variety of purposes, both for direct use as well as for integration in other AI systems;
For the example of LLM’s used in a healthcare environment, these are generally based on GPAI models and tailored to serve a specific healthcare purpose, for example, summarizing healthcare data for inclusion in an Electronic Health Record. As such, similarly to low-risk products, Article 50 of the AI Act applies and providers of these systems need to ensure that:
The AI system generating synthetic audio, image, video or text content, shall ensure that the outputs of the AI system are marked in a machine-readable format and detectable as artificially generated or manipulated; and
Providers shall ensure their technical solutions are effective, interoperable, robust and reliable as far as this is technically feasible.
LLM under FDA’s framework
To date no devices using LLM (or GPAI) have been cleared in the United States as Medical Devices. We will have to wait and what FDA expects once the first de Novo or PMA clearance will be published, e.g. in terms of the classification of such devices and the specific requirements. In any case, it will be safe to assume ‘special controls’ will be put in place should LLM supported AI systems be covered under the 510(k) regime.
When a LLM is not regulated as a medical device, and does not fall under the definition of a medical device, there are no specific regulatory requirements that apply to the use of the LLM.
Conclusions
Large language models clearly introduce opportunities for use in healthcare systems, they have the potential to increase efficiency and lower administrative costs. At the same time, the use of these models does not come without risk, incorrect information may lead to patient harm as has been demonstrated in scientific research.
If these models perform a function that fits the definition of a medical device, they are likely to be regulated as medical devices, where they are classified as High-Risk AI systems under the AI Act, and consequently, they will be strictly regulated.
At the same time, where these models do not perform a function that fits the definition of a medical device, they fall outside of the scope of the medical devices regulations and similarly also outside of the scope of being regulated as High-Risk under the AI Act (with some exceptions as clarified in the text). Instead they will be regulated as GPAI Systems under the AI Act, where minimal requirements apply to such systems.
When looking at the future, the Electronic Health Data Space Regulation is making its way to being published in the near future. The proposed regulation will regulate Electronic Health Record (EHR) Systems and enforce development (verification and validation testing), and meeting essential requirements regarding interoperability and cybersecurity requirements, along with the requirement for CE marking of such systems. The definition of EHR systems will include systems that convert or edit electronic health records, and could easily include systems that embed LLM to perform such functions.
Until the above frameworks are in place, it is recommended for any manufacturer that designs and develops AI systems that fall outside of the scope of the medical device regulations to consider the general standards for healthcare software, including the IEC 82304-1 regarding the validation of such systems, IEC 81001-5-1 regarding the cybersecurity management and ISO 14971 for risks management purposes as referred to in IEC 82304-1 and IEC 81001-5-1.