AI Act Explained: Navigating Data Governance in the Age of Artificial Intelligence
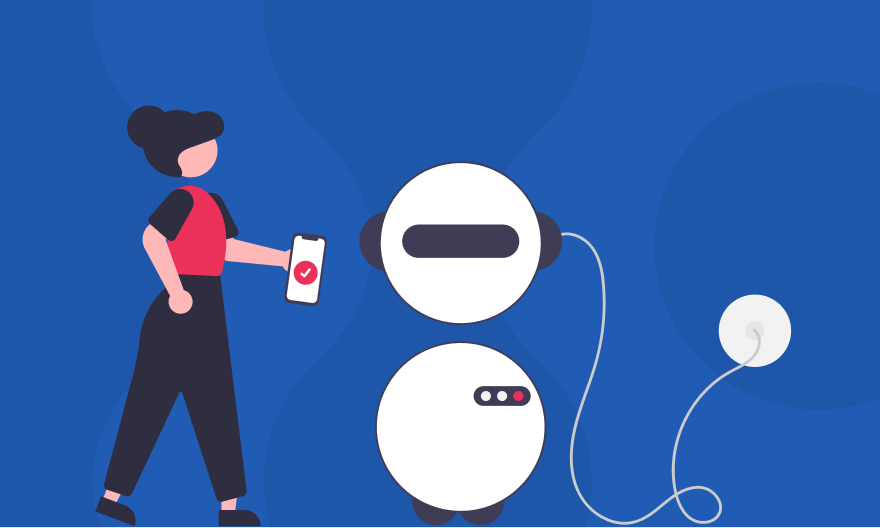
Dive into the AI Act with our comprehensive overview, designed to guide you through the complexities of data governance in the AI era. Understand the regulatory frameworks, compliance strategies, and best practices for managing AI-driven data securely and ethically. Perfect for businesses and professionals seeking to align with the latest standards in artificial intelligence governance.
After 3 years of development, it seems that a final version of the AI Act is now publicly available since it was recently published (leaked) on LinkedIn. This version gives us a perspective of where the AI Act may finally be headed. It is worth mentioning that the version that was leaked contains some obvious and some less obvious mistakes and should be read with a degree of caution.
Some of the first impressions of the text give us insight into the agreements established between the European Commission, the European Council and the European Parliament. Underneath we present the three four key take-aways that are worth calling out based on the first assessment:
The proposal of the European Parliament to transpose the requirements from the AI Act straight into the MDR & IVDR (amongst others from Annex II Section A) has not been agreed, instead an alternative agreement was made, which clarifies that manufacturers can demonstrate compliance through their existing documentation and procedures. This means for example, addressing risks regarding AI in existing risk management frameworks, adding quality procedures to their existing quality management system and so on (recital 63);
Organizations that are considered SME’s and start-ups will be able to provide elements of the technical documentation in a simplified manner, namely through a form which is to be developed by the European Commission (Article 11.1). It is unclear how such simplified manner would be compatible with the established frameworks for example for medical devices;
The conformity assessment procedures executed for AI-systems, will be integrated into existing conformity assessment procedures. Effectively, that means for organizations already subjected to Notified Body assessment, would still be subjected to the same assessment, however that assessment would also consider the requirements from the AI Act. At the same time, the Notified Body will need to comply with the requirements set out in the AI Act in order to integrate such assessment.
The definition proposed by the European Parliament for significant risk was not agreed on, which would have introduced a new risk definition different from the other New Legislative Framework regulations. In addition, the requirements from Article 9 on risk management have removed the requirement to explicitly assess risks to the environment. The understanding is that this is removed since a high level of protection of the environment is a fundamental right (recital 28a), against which risk management anyway needs to be conducted.
There is much more to unpack in the final text, as more analysis will become available. For the rest of this blogpost we aim to focus on the aspect of Data Governance, which is already aligned with the latest consolidated text of the AI Act.
Data is the foundation of Artificial Intelligence models. The use of data to develop Artificial Intelligence System differentiates these models from traditional heuristics based computer systems. The definition of Artificial Intelligence as set out by the AI Act and based on the OECD definition reads as follows:
“An AI system is a machine-based system that, for explicit or implicit objectives, infers, from the input it receives, how to generate outputs such as predictions, content, recommendations, or decisions that [can] influence physical or virtual environments. Different AI systems vary in their levels of autonomy and adaptiveness after deployment,”
AI systems require data for their development, testing and validation purposes. Once in use AI systems require input data to infer outputs. AI systems may be programmed to continue to learn after being taken into use.
Due to the explicit nature of AI Systems, it is not surprising that the AI Act introduces new, extensive requirements around the use of data for AI Systems. At the same time, the Data Act and Data Governance Act have been introduced as part of the larger European Strategy for Data. In addition, the European Strategy also set out the development of the European Health Data Space Regulation (EHDS). All these legislative documents complement each other, and should be read in parallel to grasp the full understanding of Data Governance.
As an interesting note, the EHDS which is under development will introduce a waiver for the secondary use of healthcare data for the purposes of training, validating and testing of AI algorithms for commercial purposes. Obviously, the legislation will introduce controls to ensure that such activities will not compromise the rights and freedoms of European citizens.
What does Data Governance mean under the AI Act?
Data governance refers to the practices that can be followed to ensure a high level of data ‘quality’ and ‘privacy’ throughout the data lifecycle. Specifically, high data quality requirements may not go hand in hand with privacy. To illustrate this, we will use an example from the healthcare field.
“An algorithm that was commonly used to identify patients who benefit most from high-risk care management showed to be highly biased against African Americans by predicting future health care costs rather than actual health needs—this choice of outcome introduced systematic bias to the model and could have led to the denial of optimal care for millions of African Americans by making them less likely to be enrolled in high-risk medical care programs” (Obenmeyer, 2019).
To detect such biases after the release of an AI System onto the market through monitoring, would require organizations to systematically gather the ethnicity and race of patients. Such processing of information is prohibited by privacy law, unless there is a proper legal basis to do so. We will examine the needs and implications of data governance to achieve high quality data whilst at the same time preserving privacy.
Data Quality under the AI Act
The AI Act requires organizations that train, validate and test AI systems using data that such data is of sufficiently high quality. In the initial draft of the AI Act set out by the European Commission, the initial thinking resulted in overly strict requirements, for example, the note that datasets (used for training, validation and testing) should be ‘free of errors’. This was softened by the European Council by adding the wording ‘to the best extent possible’, and later proposed by the Parliament (amendment 288) for changing into ‘appropriately vetted for errors’. The ‘appropriately vetted for errors’ has been removed from the final consolidated version, and the main wording of the European Council has made it in.
Article 10 for High Risk AI explains the requirements around data governance, albeit it should be mentioned that the text remains rather vague (‘practices shall concern’). It doesn't exactly clarify what is meant by those practices, such as 10.2(b) ‘The relevant Design Choices’’. Annex IV, listing the needs for technical documentation, 2(d) clarifies further that such measures shall include the documentation thereof. Article 17 requires developers of high-risk AI systems to implement procedures which support data governance:
“(f) systems and procedures for data management, including data acquisition, data collection, data analysis, data labelling, data storage, data filtration, data mining, data aggregation, data retention and any other operation regarding the data that is performed before and for the purposes of the placing on the market or putting into service of high-risk AI systems;”
In summary, data governance will require organizations to implement strong procedures (per Article 10 & 17), and evidence of the execution of such procedures may need to be stored as part of the AI system’s technical documentation (per Annex IV). As an organization developing an AI system which may be classified as high-risk under the AI Act, you will need to start implementing procedures and documentation within your organization to ensure that you can demonstrate with the AI Act in the future. The below overview provides indications of what type of procedures and technical documentation you could start to implement to evidence compliance.
Article | Requirement | Technical Documentation | Quality Management System |
---|---|---|---|
10.2 | Transparency with regards to the original data collection purpose, Data preparation processes, Data assumptions, Data availability, quantity and suitability, Bias examination, Methods to detect, prevent and mitigate bias, Gaps within data | Include a ‘data management plan(s)’ & ‘data management report(s)’ within the technical documentation for pre-market purposes and a include data aspects in the ‘post market monitoring plan’ | Ensure that ‘design and development procedures’ and ‘post market monitoring procedures’ identify the needs for preparing ‘data management plans’ and ‘data management report(s)’. |
17.1 (f) | ‘Systems and procedures for data management, including data acquisition, data collection, data analysis, data labelling, data storage, data filtration, data mining, data aggregation, data retention and any other operation regarding the data that is performed before and for the purposes of the placing on the market or putting into service of high-risk AI systems;’ | Ensure that within ‘data management plan(s)’ and ‘data management report(s)’ it is explained what actions are performed on data before it was used for the intended purpose of the data | Ensure that the Quality Management System includes procedures for ‘data management’ (such as acquisition, storage, changes, retention, DPIA’s) and for ‘data processing’ (such as data analysis, bias detection) |
Data Privacy under the AI Act
Obviously organizations who collect, store and process personal data, for whatever reason, are bound to the requirements set out in the GDPR 2016/679. Amongst others the regulation (the GDPR) requires a valid basis for the collection of personal data (per article 6) and as applicable an additional basis per article 9 for special categories of personal data (e.g. racial or ethnic origin, data concerning health etc).
Specifically around data privacy, the AI Act may introduce a conflict (article 10.2(f)) with the GDPR, e.g. the AI Act requires organizations to assess potential risks for biases both during the development of the AI system as whilst the AI system is on the market. Note that specifically the prohibition of processing ethnicity can introduce complexity, since it is well known that many AI algorithms are trained on datasets that lack diversity. The GDPR explicitly prohibits the processing of special categories of personal data, unless one of the specified exceptions apply (article 9.2).
The GDPR specifies under Article 9.2(g)
“(g) processing is necessary for reasons of substantial public interest, on the basis of Union or Member State law which shall be proportionate to the aim pursued, respect the essence of the right to data protection and provide for suitable and specific measures to safeguard the fundamental rights and the interests of the data subject;”
To facilitate the collection of data in pre- and post-market settings, specifically where the collection of special categories of personal data would be required, the European Commission developed the following clause (Article 10.5 of the AI Act):
‘To the extent that it is strictly necessary for the purposes of ensuring bias, detection and correction in relation to the high-risk AI systems in accordance with the second paragraph, point f and fa, the providers of such systems may exceptionally process special categories of personal data referred to in Article 9(1) of Regulation (EU) 2016/679, Article 10 of Directive (EU) 2016/680 and Article 10(1) of Regulation (EU) 2018/1725, subject to appropriate safeguards for the fundamental rights and freedoms of natural persons.’
Note that whilst the AI Act may require organizations to assess bias, and the above grants some freedom to the secondary use of data for that specific purpose, it comes with extensive requirements that will need to be met (e.g. data may need to be pseudonymised, may need to be done within the boundaries of the organization, and will require strong technical security measures). In addition, it is questionable how at ease data subjects will be with organizations asking for consent to collect their ethnicity or health data (or any other special category of data).
Similar to the section about Quality above, organizations will need to embed and document how they fulfill privacy requirements, and document their considerations within the quality management system, technical documentation and their post market monitoring plans (per article 61). Such documentation will require organizations to clarify how data was collected, for which purposes the data is used and how such data is protected from unauthorized access or use. Obviously a Data Protection Impact Assessment may be of support to an organization.
The below overview provides a basis for documentation to describe how to integrate data privacy considerations.
Article | Requirement | Technical Documentation | Quality Management System |
---|---|---|---|
10.5 | To the extent that it is strictly necessary for the purposes of ensuring bias, detection and correction in relation to the high-risk AI systems | Within the ‘data management plan(s)’ & ‘data management report(s)’ and within potential ‘data protection impact assessments’ ensure a clear description of the source of the data, legal basis for accessing the data, and any needs for processing special categories of data. | Ensure that the ‘Data Management’ procedure(s) set out clear requirements for documenting the information needed for the Technical Documentation, including when execution of a Data Protection Impact Assessment may be required |
Documentation under the AI Act
Within the previous sections, we provide examples for demonstrating compliance throughout the quality management system (i.e. updating existing procedures and adding additional procedures) and within the technical documentation. The overview below provides more specific considerations for each of these documents.
Document | Type of documentation | To consider |
---|---|---|
Data Management Plan (may be updated throughout development process) | Technical Documentation per Annex IV | Description(s) incl. Initial data acquisition purpose, Data collection methods including data minimisation, Needs and characteristics, DPIA reference or description of legal basis, Bias examination plan, Data assumptions, Preparation plan, Quality testing plan, Limitations and mitigation plan |
Data Management Report (Delivered at the end of development) | Technical Documentation per Annex IV | Characteristics, Identified biases and mitigation, Completeness, Quality, Limitations, Lifecycle and maintenance process, Storage, security and retention policy |
Data Management procedure | Quality Management System per Article 17 | Acquisition process, Management plan & report references, Design and development references, Security and access restrictions, Ownership and responsibilities, Retention policy, Post market data maintenance |
Data Processing procedure (or work instruction) | Quality Management System per Article 17 | Assessment of data Quality, Standard data cleaning process, Pseudonymisation and anonymisation techniques, Bias examination techniques, Data preparation techniques |
Post Market Monitoring plan | Quality Management System per Article 17 & Article 61 | Data maintenance, Bias monitoring, Model updating |
Data Protection Impact Assessment | Quality Management System per article 10.5 & GDPR | Data acquisition legitimacy, Data subjects, Consent procedures or waiver for bias monitoring, Privacy impact assessment |
Without having clear guidance available within the European Union and with the absence of harmonized standards, the overview above will require adjustments as the final text becomes available of the AI Act, harmonized standards become published and when guidance is being made available (e.g. as set out in Article 61).
Standardization activities under the AI Act
Within the standardization request (M/593) set out by the European Commission towards CEN/CENELEC (JTC21), the European Commission asks specifically for the development of standards to address data governance and for data governance to be addressed within a standard for quality management.
Quality Management under the AI Act
Within ISO/IEC (SC42) standards have been developed for both purposes and a number of standards are still under development. ISO/IEC 42001 aims to address data management by allowing organizations to implement controls specified in Annex A. The controls in Annex A are, however, fairly high-level and fail to address the level of detail set out in the AI Act. Consequently, this standard may not be very useful towards demonstrating compliance against the AI Act. For example, it requires organizations to address data quality by:
‘The organization shall define and document requirements for data quality and ensure that data used to develop and operate the AI system meet those requirements.‘
When implementing such a control, it hardly provides for any more detail (if any) than what is required under the AI Act. Consequently, it remains to be seen whether the ISO/IEC 42001 would be useful for demonstrating compliance against the AI Act in the future.
Data governance under the AI Act
Whilst the ISO/IEC 42001 has just been published, additional standards are under development within ISO/IEC to address data governance. The ISO/IEC 5259 series (around data quality for analytics and machine learning) aims to provide more detail as to how to govern data. An overview of the standards is provided below.
Number | Title | Status |
---|---|---|
5259-1 | Overview, terminology, and examples | FDIS |
5259-2 | Data quality measures | DIS |
5259-3 | Data quality management requirements and guidelines | FDIS |
5259-4 | Data quality process framework | FDIS |
5259-5 | Data quality governance framework | DIS |
5259-6 | Visualization framework for data quality | CD |
These standards go into greater depth than the ISO/IEC 42001 and provide for more practical guidance related to data governance. For example, the ISO/IEC 5259 part 3 specifies the needs for defining the ‘Data Quality Management Lifecycle’, having ‘Data Specifications’, ‘Data Quality Plans’, ‘Data Change Management Procedures’. Part 2 provides useful measures (e.g. accuracy, completeness, consistency, credibility, currentness, etc) that can be applied to assess and describe the quality of data and Part 4 specifies considerations with regards to what could be included in processes around data management (e.g. data quality planning, data quality evaluation, data quality improvement, data quality process validation).
None of these standards are published today, however it is fair to assume that the FDIS versions of these standards will be published soon with a limited number of changes. While these standards are typically not prescriptive by nature, they can be useful as guidance for defining data quality processes and procedures, data quality assurance tests, and defining the needs for technical documentation.
Conclusions of Data Governance under the AI Act
Data is key for Artificial Intelligence systems, as such it is no surprise that the AI Act requires organizations to address data management throughout their processes, documented procedures as part of the Quality Management System and within their Technical Documentation.
If you are an organization who develops systems using (high-risk) AI, this is the moment to start documenting processes, procedures and technical documentation. Ensure that you meet at least the needs set out by the AI Act today in the proposal, and start thinking about more granular detail in those documents as explained within the ISO/IEC 5259 standards series. At any time carefully balance the need for data acquisition, securing information and processing against privacy requirements set out in the GDPR, and consider potential bias risks within data.
Having robust data governance methods implemented will support transparency and trustworthiness, both crucial aspects for building strong AI systems, demonstrating compliance with regulations and being a responsible organization.